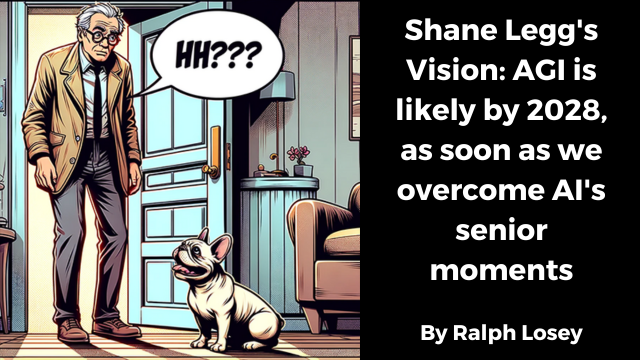
In the rapidly evolving landscape of artificial intelligence, few voices carry as much weight as Shane Legg, Founder and Chief AGI Scientist at Google DeepMind. AGI, Artificial General Intelligence, is a level of machine intelligence equal in every respect to human intelligence. In a recent interview by Dwarkesh Patel, Shane Legg talks about what AGI means. He also affirms his prior prediction that there is a fifty percent (50%) chance that AGI will be attained in the next five years, by 2028. AGI is not the same as The Singularity, where AI exceeds human intelligence, but the step just before it. Shane Legg also explains, in simple terms, the changes in current generative AI architecture that he thinks will need to take place for his very optimistic prediction to come true. The problem concerns episodic memory lapses, to which I can easily relate. Yes, if AI can just overcome its senior moments, it will be a smart as us!
Future AI chat image by Ralph Losey using his new Visual Muse GPT
Who Is Shane Legg?
Image of Shane Legg by Ralph Losey
using Photoshop AI
Shane Legg, age 42, is originally from New Zealand. He founded DeepMind in 2010 with Demis Hassabis, and Mustafa Suleyman. Deep Mind is a famous British-American AI research laboratory that specialized in neural network models. Elon Musk was one of its early investors. DeepMind was purchased by Google in 2014 for over $500 Million. DeepMind made headlines in 2016 after its self-taught AlphaGo program beat a human professional Go player Lee Sedol, a world champion.
As the Chief AGI Scientist at Google DeepMind Shane Legg is a key figure in the world of artificial intelligence . More than twenty years ago, influenced by Ray Kurzweil’s book, The Age of Spiritual Machines (1999), a book that I also read then and admired, Legg first estimated a 50% chance of achieving human-level machine intelligence by 2028. Legg’s foresight led him to return to school for a Ph.D. at the Dalle Molle Institute for Artificial Intelligence Research in 2008, where his thesis, Machine Super Intelligence, won widespread acclaim in AI circles. Shane Legg sticks by his prediction in the October 26, 2023, interview by Dwarkesh Patel reported here, even though it is now only five years away.
Legg’s role at DeepMind has primarily focused on AGI technical safety, ensuring that when powerful AI systems are developed, they will align with human intentions and prevent potential catastrophes. His optimism about solving these safety challenges by 2028 reflects his belief in the feasibility and necessity of aligning AI with human values. Despite recent public skepticism and concerns about the dangers of AI, Legg remains a strong advocate of the positive potential of AI.
Understanding the Basic Architectural Design Needed for AGI
In the excellent video interview by Dwarkesh Patel on October 26, 2023, Shane describes in simple terms the basic architecture of LLMs and the main problem with what he calls episodic memory. He explains that this the main obstacle preventing LLM’s from attaining AGI human level of intelligence. Recall how many of us have been complaining about the small size of the input, or context window, as Leggs put it, of ChatGPT. See eg. How AI Developers are Solving the Small Input Size Problem of LLMs and the Risks Involved (June 30, 2023). The input window is where you submit your prompts and particular training instructions or documents to be studied and summarized. It is too small for most of the AI experiments that I have done and forces workarounds, such as use of summaries instead of full text. This in turn leads causes the AI to forget the original prompts, leading to AI mistakes and hallucinations. Poor AIs with severe episodic memory problems. It certainly triggers my empathy brain centers.
Image by Ralph Losey using MidJourney of sad, forgetful AI. Not nearly as smart as us.
In the interview Shane Legg explains the gap between short term memory training, with limited size prompt, and long-term training memory, where the LLMs are loaded with trillions of words. This gap between the small, short term information ingestion, and the large, lifetime input of information, mirrors the processes and gaps of the human brain. According to Legg, this gap, which he refers to as episodic memory, is the key problem faced by all LLMs today. Unlike humans, generative AI does not have much in the way of episodic memory. The gap is too big, the bridge is too small. It is the main reason generative AI cannot yet reach our level of intelligence. Shane Legg is not too happy about the episodic gap and wants to bring AI up to our level as soon as safely possible, which, again, he thinks is likely anytime in 2028 or shortly thereafter,
Image of happy Shane Legg robot by Ralph Losey using Photoshop AI (click to see AI video)
Here is Shane Legg’s explanation.
The models can learn things immediately when it’s in the context window and then they have this longer process when you actually train the base model and that’s when they’re learning over trillions of tokens. But they miss something in the middle. That’s sort of what I’m getting at here.
I don’t think it’s a fundamental limitation. I think what’s happened with large language models is something fundamental has changed. We know how to build models now that have some degree of understanding of what’s going on. And that did not exist in the past. And because we’ve got a scalable way to do this now, that unlocks lots and lots of new things.
Now we can look at things which are missing, such as this sort of episodic memory type thing, and we can then start to imagine ways to address that. My feeling is that there are relatively clear paths forward now to address most of the shortcomings we see in the existing models, whether it’s about delusions, factuality, the type of memory and learning that they have, or understanding video, or all sorts of things like that. I don’t see any big blockers. I don’t see big walls in front of us. I just see that there’s more research and work and all these things will improve and probably be adequately solved.
YouTube video of interview, quotes at 0:4:46 – 0:6:09
The big problem is with intermediate memory and learning, but Patel thinks this problem is fixable. He comes back to this key issue later in the interview. What he does not mention, and perhaps may not know, is that most humans have the same type of episodic, step by step, medium-term memory problem. That’s why the opening and closing statements in any trial are critical. Juries tend to forget all the evidence in between.
Robot making closing argument to jury. Image by Ralph Losey using Visual Muse
That is also the reason most professional speakers use the tell, tell and tell approach. You start by telling what you will say, hopefully in an intriguing matter, then you tell it, then you end with a summary of what you just said. Still, Shane Legg thinks, hopefully not too naively, there is a way to copy the human brain and still overcome this intelligence problem.
Here is the next relevant excerpt from Legg’s interview.
[T]he current architectures don’t really have what you need to do this. They basically have a context window, which is very, very fluid, of course, and they have the weights, which things get baked into very slowly. So to my mind, that feels like working memory, which is like the activations in your brain, and then the weights are like the synapses in your cortex.
Now, the brain separates these things out. It has a separate mechanism for rapidly learning specific information because that’s a different type of optimization problem compared to slowly learning deep generalities. There’s a tension between the two but you want to be able to do both. You want to be able to hear someone’s name and remember it the next day. And you also want to be able to integrate information over a lifetime so you start to see deeper patterns in the world.
These are quite different optimization targets, different processes, but a comprehensive system should be able to do both. And so I think it’s conceivable you could build one system that does both, but you can also see that because they’re quite different things, it makes sense for them to be done differently. I think that’s why the brain does it separately.
YouTube video of interview, quotes at 0:4:46 – 0:6:09 and 0:12:09 – 0:13:21.
Brain architecture balance image by Ralph Losey using his Visual Muse GPT
Shane’s analysis suggests that mimicking this dual capability of human learning is key to AGI. It’s about striking a balance: rapid learning for immediate, specific information and slow learning for deep, general insights. The current imbalance, with the time gaps not fully bridged, is the weak point in AI architectures.
Since generative AI design is based on human brain neurology, this weakness is hardly surprising. Ask any senior, unlike young PhDs we are all very familiar with episodic memory gaps. Now why did I come into this room? We can and do even laugh about it.
Man having a funny senior moment with his dog. Image by Ralph Losey using Visual Muse
Such self-awareness, much less humor, is not even considered to be intelligence by the AI scientists and so is not part of any of the AGI tests under development. Yet, it is obvious commonsense that there is much more to being a human than mental IQ. This is yet another reason I favor a close hybrid merger of AGI with human awareness. We need to keep the pure intellect of machines grounded by direct participation in human reality. See eg: Pythia: Prediction of AI-Human Merger as a Best Possible Future (November 9, 2023), comment-addendum, and Circuits in Session: Analysis of the Quality of ChatGPT4 as an Appellate Court Judge (Conclusion) (November 1, 2023). When an AI can truly laugh and feel empathy, then it will pass my hybrid multimodal AGI Turing test.
The Big Prediction: 50% Chance of AGI by 2028
Right after making these comments about changes in architecture needed to make AGI a reality, Shane Legg predicts, once again, that there’s a 50% chance AGI as he understands it will be achieved by 2028. He does not say that Google Mind will be the first company to do that, but certainly suggests it might be them, that they are close, but for the mentioned gaps in episodic memory.
I think there’s a 50% chance that we have AGI by 2028. Now, it’s just a 50% chance. …
I think it’s entirely plausible but I’m not going to be surprised if it doesn’t happen by then. You often hit unexpected problems in research and science and sometimes things take longer than you expect.
YouTube video of interview at 0:36:34 and 0:37:04.
Shane goes on to say he does not see any such problems now, but that you never know for sure what unforeseen roadblocks may be encountered, and thus the caveats.
Surreal Roadblocks image by Ralph Losey using his Visual Muse GPT
The Evolution and Impact of AI Models
Next in the interview Shane Legg predicts the improvements that he expects will happen to generative AI up until the time that full AGI is attained.
I think you’ll see the existing models maturing. They’ll be less delusional, much more factual. They’ll be more up to date on what’s currently going on when they answer questions. They’ll become multimodal, much more than they currently are. And this will just make them much more useful.
So I think probably what we’ll see more than anything is just loads of great applications for the coming years. There can be some misuse cases as well. I’m sure somebody will come up with something to do with these models that is unhelpful. But my expectation for the coming years is mostly a positive one. We’ll see all kinds of really impressive, really amazing applications for the coming years.
YouTube video of Shane Legg interview at 0:37:51.
Image of coming improvement to GPT by Ralph Losey using Visual Muse
Dr. Legg at least recognizes in his otherwise glowing predictions that someone might come up with “something to do with these models that is unhelpful.” He seems blissfully unaware of the propaganda problems that misaligned, weaponized generative AI have already caused. Or, at least, Shane Legg choose not to bring it up. It was, after all just a short interview and those types of tough questions were not asked.
Instead, Shane focused on positive predictions of rapid improvements in accuracy and reliability. He pointed out that such improvements are crucial, especially in applications where precision and factuality are paramount, such as in medical diagnosis and legal advice. The noted the shift towards multimodality is equally significant. Multimodal AI systems can process and interpret various forms of data – like text, images, and sound – simultaneously. This capability will vastly enhance the AI’s understanding and interaction with the world, making it more akin to human perception.
Hybrid AI Human judges on future. Image by Ralph Losey using Visual Muse
Conclusion
Dr. Shane Legg is one of the world’s leading experts in AI. His predictions about achieving AGI by 2028, with a 50% probability, should be taken seriously. As bizarre as it may seem, in view of the many stupid errors and hallucinations we now encounter with generative AI, human level computer intelligence in every field, including law, could soon be a reality. To be honest, I would hedge my prediction to something less than fifty percent, but Shane Legg is one of Google’s top scientists and, apparently, does not suffer from the same episodic memory gaps that I do. Young Dr. Legg has teams of hundreds of the world’s top AI scientists reporting to him. His insights and optimistic predictions of AGI should be taken seriously, no matter how far out they may seem. Let this blog serve as the first tell, listen to the entire YouTube video of Shane Legg interview as the second, and then tell yourself your own conclusions. That kind of episodic learning may well be the essence of true human intelligence.
Digital punk art image of AI lab using Visual Muse GPT by Ralph Losey.
Legg’s explanation of how the human mind works, and how we learn and remember, seems right to me. So does his focus on the episodic memory problem. The hybrid multimodal approach patterned after our own brain structures appears destined to replicate our measurable intelligence soon, be in 2028 or beyond. Then the even more interesting challenge of guiding the super-intelligence will follow. That will lead to a truly hybrid, fully connected, human-machine experience. See: Pythia: Prediction of AI-Human Merger as a Best Possible Future, (November 9, 2023); New Pythia Prophecy of AI Human Merger in Traditional Verse (November 14, 2023); ChatGTP-4 Prompted To Talk With Itself About “The Singularity” (April 4, 2023); and, Start Preparing For “THE SINGULARITY.” There is a 5% to 10% chance it will be here in five years. Part 2 (April 1, 2023).
Shane Legg’s vision of the evolving capabilities of AI models give us hope for that future, not doom and gloom. He may be right. Do what you can now to be ready to plug in and leap forward.
Digital punk art plugged-in image using Visual Muse GPT by Ralph Losey.