De-identification of data refers to the process used to prevent personal identifiers—both direct and indirect—from being connected with information. The EU General Data Protection Regulation (“GDPR”), which will replace the Data Protection Directive 95/46/EC effective May 25, 2018, is clear that it does not apply to data that “does not relate to an identified or identifiable natural person or to data rendered anonymous in such a way that the data subject is not or no longer identifiable.”1 Anonymization of personal data refers to a subcategory of de-identification whereby direct and indirect personal identifiers have been removed and technical safeguards have been implemented such that data can never be re-identified (e.g., there is zero re-identification risk). This differs from de-identified data, which may be re-linked to individuals using a key—a code, algorithm, or pseudonym. The GDPR defines pseudonymization as “the processing of personal data in such a way that the data can no longer be attributed to a specific data subject without the use of additional information, provided that such additional information is kept separately and is subject to technical and organizational measures to ensure that the personal data are not attributed to an identified or identifiable natural person.”2 Therefore, under the GDPR, Pseudonymous data refers to a data from which identifiers in a set of information are replaced with artificial identifiers, or pseudonyms, that are held separately and subject to technical safeguards.
Although pseudonymization is not alone a sufficient technique to exempt a controller from the GDPR requirements, the GDPR recognizes that pseudonymization “can reduce risks to the data subjects concerned and help controllers and processors meet their data-protection obligations.”3 Accordingly, the GDPR creates significant incentives for controllers to pseudonymize personal data. Under the GDPR, pseudonymization can help a controller: (1) fulfill its data security obligations; (2) safeguard personal data for scientific, historical, and statistical purposes; and (3) mitigate its breach notification obligations.
Pseudonymization can help a controller fulfill its data security obligations. The GDPR requires that controllers implement reasonable and appropriate “technical and organizational measures, such as pseudonymization” to protect data by design and by default.4 Furthermore, the GDPR requires that controllers implement reasonable and appropriate “technical and organizational measures” to ensure data security.5 Pseudonymization is one of two examples that the GDPR provides for explicitly as an example of a security measure that can help a controller meet its data security obligations.
Pseudonymization can help a controller safeguard personal data for scientific, historical, and statistical purposes. The GDPR requires that data only be processed for the limited purpose for which it was collected, but provides an exception to this purpose limitation for data processing for scientific, historical, or statistical purposes provided “appropriate safeguards” are implemented.6 The GDPR explicitly provides that pseudonymization is a safeguard that can help a controller meet its “appropriate safeguards” requirement to process data for scientific, historical, and statistical purposes.
Pseudonymization can help a controller mitigate its breach notification obligations. Under the GDPR, controllers must notify government regulators if there is a “risk to the rights and freedoms of natural persons,” and consumers if there is a “high risk to the rights and freedoms of natural persons.”7 As pseudonymization is a risk-based safeguard that can reduce risks to data subjects, controllers that have implemented pseudonymization may be able to avoid notification obligations under the GDPR.
Anonymization is not a single technique, but rather a collection of approaches, tools, and algorithms that can be applied to different kinds of data with differing levels of effectiveness. In 2014, the Article 29 Working Party (WP29) released its Opinion 05/2014 on Anonymization Techniques8 that examines the effectiveness and limits of various anonymization techniques against the legal framework of the EU. The opinion states that anonymization results in processing personal data in a manner to “irreversibly prevent identification.”9 The WP29 identifies the following seven techniques that can be used to anonymize records of information:
-
Noise Addition: The personal identifiers are expressed imprecisely (e., weight is expressed inaccurately +/- 10 lb).
-
Substitution/Permutation: The personal identifiers are shuffled within a table or replaced with random values (e., a zip code of 80629 is replaced with “Magenta”).
-
Differential Privacy: The personal identifiers of one data set are compared against an anonymized data set held by a third party with instructions of the noise function and acceptable amount of data leakage.
-
Aggregation/K-Anonymity: The personal identifiers are generalized into a range or group (e., a salary of $42,000 is generalized to $35,000 - $45,000).
-
L-Diversity: The personal identifiers are first generalized, then each attribute within an equivalence class is made to occur at least “l” times. (e., properties are assigned to personal identifiers, and each property is made to occur with a dataset, or partition, a minimum number of times).
-
Pseudonymization – Hash Functions: The personal identifiers of any size are replaced with artificial codes of a fixed size (e., Paris is replaced with “01”, London is replaced with “02”, and Rome is replaced with “03”).
-
Pseudonymization – Tokenization: The personal identifiers are replaced with a non-sensitive identifier that traces back to the original data, but are not mathematically derived from the original data (i.e., a credit card number is exchanged in a token vault with a randomly generated token “958392038”).
Key Definition: “Direct Identifiers” are data that identifies a person without additional information or by linking to information (e.g., name, telephone number, SSN, government issued ID).
|
Key Definition: “Indirect Identifiers” are data that identifies an individual indirectly (e.g., DOB, gender, ethnicity, location, cookies, IP address, license plate number).
|
Key Definition: “Aggregation” of data refers to the process by which information is compiled and expressed in summary form.
|
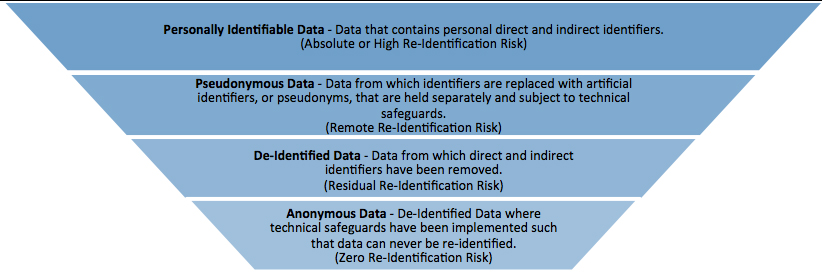
[View source.]