By now, we all know that Generative AI models are trained on massive amounts of data, much of which is collected from the Internet, as well as other sources.
And we also know that Gen AI is being used to generate massive amounts of new content, much of which is also being made available online.
So what happens when the Gen AI-generated content is fed back into the AI model as additional training data? Maybe nothing, right? Maybe the model just gets stronger because the Gen AI outputs are so fluent? After all, a lot of Gen AI output is fairly indistinguishable – to the human eye – from human-created content. And there is still way more (for now) human-created content than AI-generated content, so the Gen AI content’s impact might be expected to be negligible, at most.
But new research from a team led by Oxford professor Ilya Shumailov reveals some concerning results. Even a small amount of AI-generated data fed back into the model as training data can lead to model collapse.
The idea is explained by the diagram below (reprinted from TechCrunch):
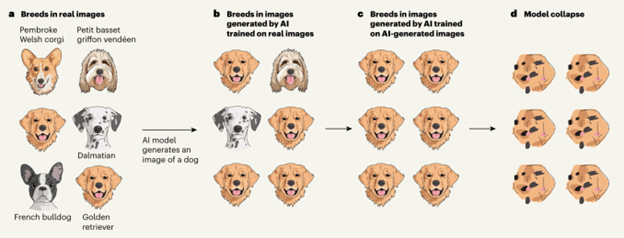
Naturally-occurring training data will have a broad distribution. If you think of a bell curve, the center of the curve is relatively low, and there are long tails leading out from the center. In the diagram above, this is represented by the variety of dog breeds represented in the first image, (a).
Generative AI comes up with an output by selecting highly probable choices. In other words, it tends to pick outputs from the middle of the bell curve. This is why they look “normal” or plausible to us. While it’s possible to tweak the settings on the Gen AI model to move a little farther out from the center of the bell curve – to be more “chaotic” or “creative” – the core idea is that Gen AI picks the common data. So in the figure above, (b) shows that Gen AI will tend to over-weight the golden retriever, because it is the most common breed in the (a) dataset. And over multiple generations of feeding the Gen AI outputs back in as new training data, golden retrievers will become more and more heavily represented, until they represent all the data. In bell-curve terms, the curve will become progressively higher and narrower until it is just a spike of all golden retrievers, (c) in the figure.
Then a strange thing happens. The AI model starts to “forget” the characteristics of its original training data, and the outputs become more nonsensical. In (d) above, instead of golden retrievers, we see abstract blobs that are perhaps more reminiscent of chocolate-chip cookie shapes than golden retrievers. The explanation for this ultimate loss of coherence is not entirely clear; it may be due to the magnification of statistical anomalies in the dataset through re-use over time. It bears noting that other teams of researchers have disputed that model collapse is inevitable, and/or have argued that the worst effects shown by the Nature article authors’ research can be mitigated by maintaining a mix of synthetic and human-created training data.
The phenomenon has been illustrated a number of different ways.
Handwritten numbers in the New York Times’ coverage:
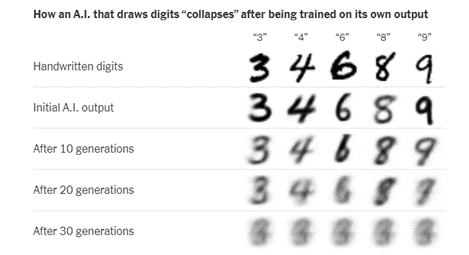
Generated text in the Nature article:
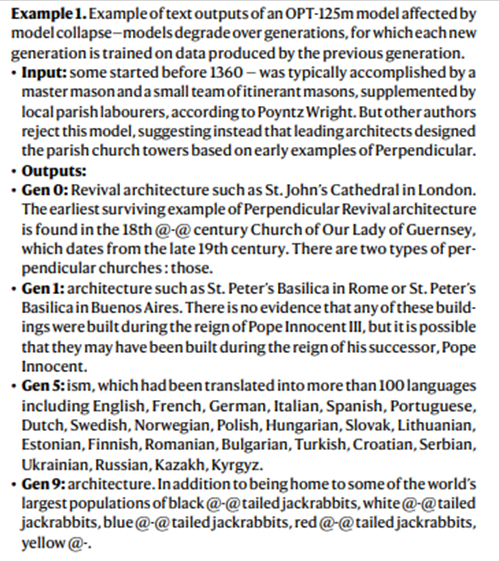
Because of the dangers to AI models posed by re-use of Gen AI outputs, the Nature article concludes, “The need to distinguish data generated by LLMs from other data raises questions about the provenance of content that is crawled from the Internet: it is unclear how content generated by LLMs can be tracked at scale.”
Small surprise, then, at the news that Open AI has expressed support for a AB3211, a proposed California law that would require watermarking on AI-generated content. In addition to performing an intended consumer-notice function, such watermarks would provide a handy way for online data scraping tools to track and exclude LLM-generated content, at scale, as they collect new training data.
[View source.]